The longer it takes to catch a defect and trace its root cause, the greater the risk it will impact your customers.
Take this example from a manufacturer of agricultural machinery. A a faulty gear system poised a serious safety issue for customers in the field. Full production was halted for several weeks until the cause could be found and addressed.
Can your operation afford this much production downtime? What about the risk to your reputation of allowing defective products to reach customers?
To discuss how Sciemetric’s process monitoring and manufacturing analytics platforms can help your team contain defects fast:
In-process testing and data analysis target defects fast
With Sciemetric, your team can visualize and characterize what a defect looks like in minutes or hours versus days or weeks.
- In-process testing (IPT) builds measurement, data collection and reporting into each step of the manufacturing process.
- Intelligent process monitoring and pass/fail determination occurs at the source, to catch defects before they can advance to the next step of production.
- Data that charts what happened to each part through every step of production and test is consolidated into a single birth history record, indexed by serial number.
- Using process signature verification, select data is converted into histograms that show the profile of a good part and a range of acceptable deviation.
Learn more about the role of digital signature analysis in defect detection and resolution.
Explore how our QualityWorX suite of data management and manufacturing analytics software can help your team with defect avoidance and boost the quality of your output.
Case study: 7 engines, instead of 10,000
An automotive OEM used its archive of data collected into Sciemetric QualityWorX to trace a defect that had never been seen before. Test signatures captured by Sciemetric test systems on the production line were called up by serial number and reviewed. This revealed an anomaly in a process signature that indicated the presence of the defect.
This left the manufacturer facing a recall of 10,000 engines. But by reviewing the historical test data for these engines, the manufacturer determined that only another six had the defect. By limiting a recall to seven instead of 10,000 engines, the manufacturer avoided a $5-million expense.
New parameters were then added to the standard test to ensure the same defect would never leave the plant again.
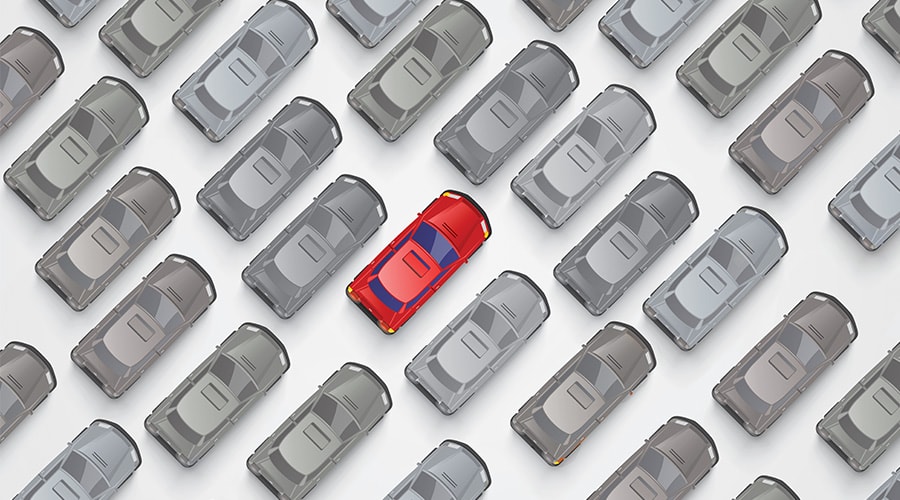
How to use production data to minimize the cost and impact of a production recall
Sciemetric developed the Ask The Experts video series to provide quick, straightforward answers to the questions our customers ask us every day. In this video, Sciemetric data expert Robert Ouellette discusses how you can use production data to minimize the cost and impact of a production recall.