Contributed by: Rob Plumridge
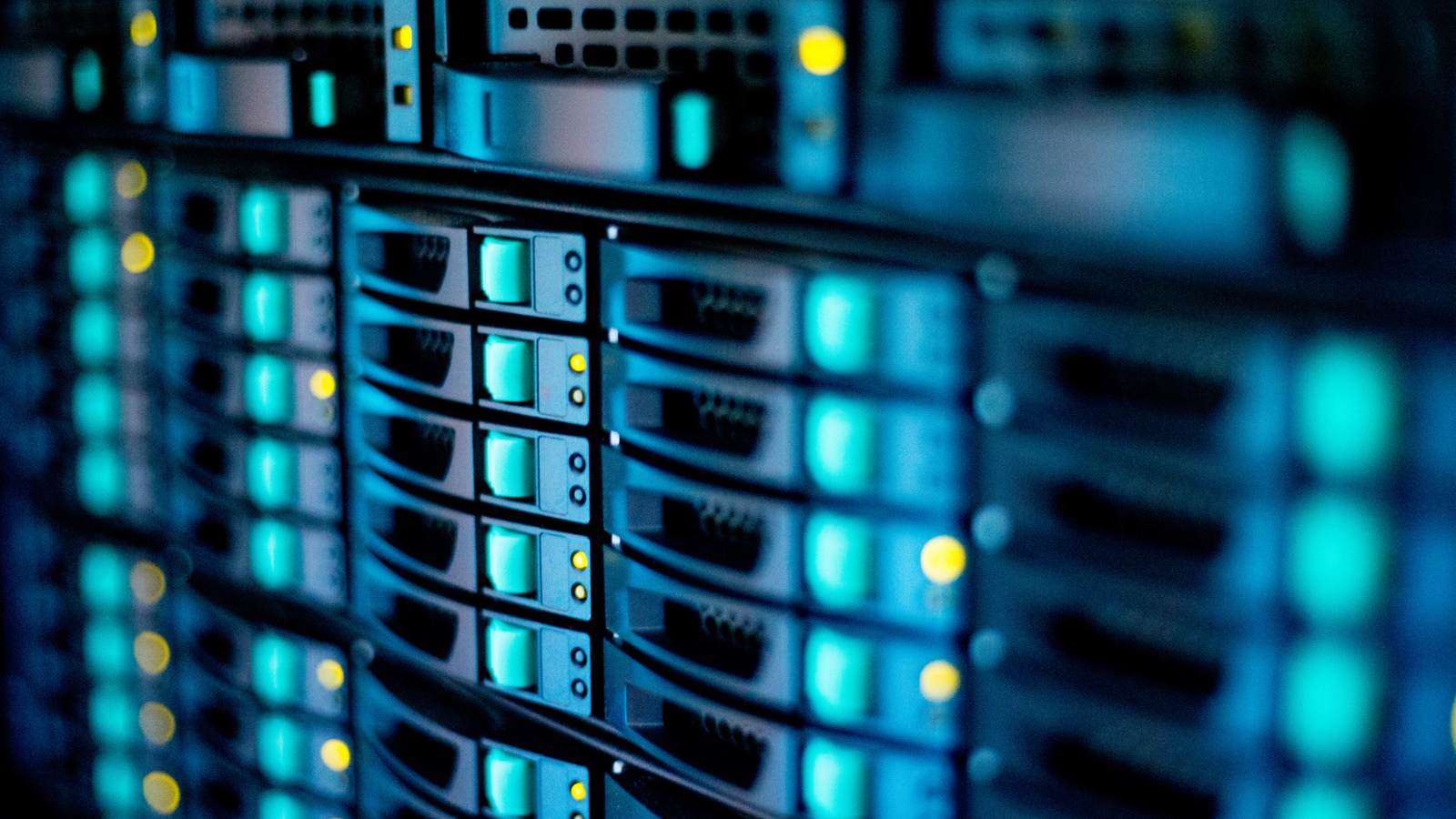
In the previous post, I talked about the need to collect and organize production data to quickly troubleshoot a bottleneck on the line with targeted root cause analysis.
But when it comes to your long-term strategy, manufacturers often ask us to clarify how much data they need to keep, what data they should archive, and for how long.
We often use the example of an engine oil pan that fails a leak test. What is the cause of the failure—a faulty gasket due to improper dispensing, an improperly installed gasket due to incorrect position, bolts that didn’t tighten down correctly, poorly machined surfaces due to excessive vibration at a machining center?
Getting to the root cause of this flaw could require investigation of a dozen or more machining, dispensing, fitting and rundown operations, each with its own dataset of feature checks. With access to the right data, this process of investigation will be fairly painless.
When starting out, the idea is to start with heavy data collection, working to collect every bit of data from every process or test that touched each part as it moved through production. As we review more data from more parts, we will gain a deeper understanding of what feature checks and limits are sufficient to distinguish a good oil pan assembly from a bad one. Using this analysis, we might see ways to now pare back on how much data we are collecting or how many different feature checks we are implementing, which gives us a better idea of what data is in your best interest to keep long-term.
When in doubt, more data is always better
While your team may only regularly review five percent of the data you collect, there is still a lot of value in retaining that other 95 percent for reprocessing. It could be to carry out “what-if analysis” for continuous process improvement, or as a hedge against future warranty claims.
With the latter, it can often be the case where borderline passes may result in a failure or performance issue down the road. The quality team doesn’t know it has an issue until the warranty claims start to come in. Because they did collect and archive that production data, they can pull up by serial number for those parts or assemblies that are subject to a warranty claim.
Targeted root cause analysis can then be carried out to see if any of these faulty units have anything in common, like a borderline pass or an anomaly on a particular feature check. If so, we can filter through the process signatures for other parts and flag any that have the same anomaly. This enables a selective recall rather than a costly mass recall. Processes on the line can also then have their limits re-adjusted to prevent the same flaw from ever slipping through again.
Identifying how long to store your data
Identifying how long to store your data depends on how long you want to support that unit in the field. How long is the warranty on your products? If you are a supplier to an OEM, how long is their warranty on their products that includes your part or assembly? Your long-term storage strategy should at very least cover those time periods.
The key here is that cost should not be your first consideration when it comes to storing your production data. Storage capacity, either on-premise or in the cloud, is affordable these days. When it comes to machine vision, various compression methods can also address file size concerns. And when the day comes that you need to conduct a root cause analysis, having that data available for a selective recall will likely be worth much more—to your bottom line and your brand reputation.
Looking to improve data collection and analysis on your production line? We can help. Contact us.