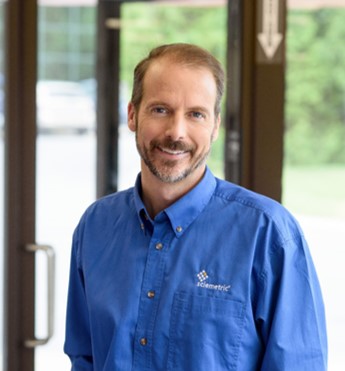
Contributed by: Richard Brine - CTO
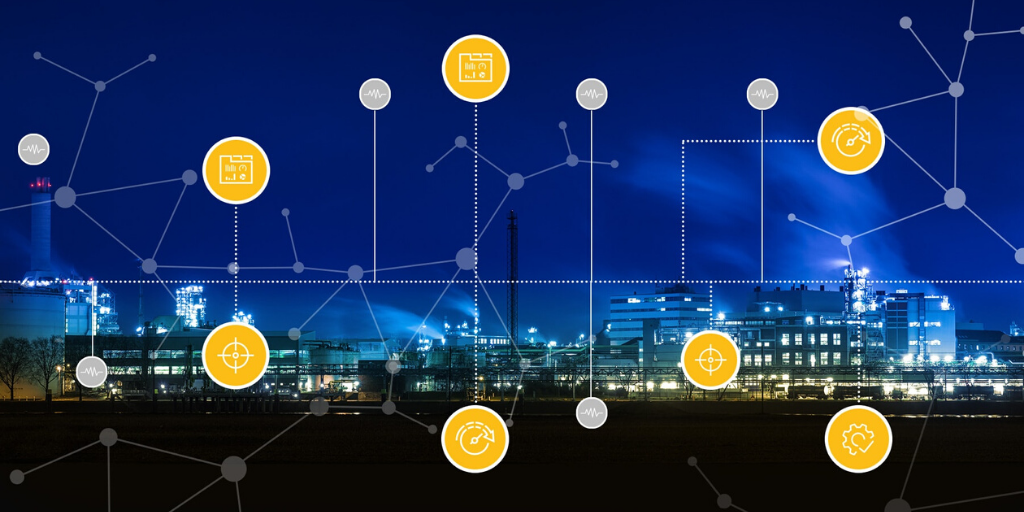
For manufacturing and industrial process control, making more effective use of data in real-time has become a necessary part of doing business to reduce costs, improve efficiencies and achieve new benchmarks for quality, performance and compliance.
Even outdated legacy equipment can now be enhanced with sensors that can monitor any number of parameters. Enabling data collection at the level of the individual machine or process is not the problem. Collecting and managing the data from hundreds or thousands of deployed sensors, on the other hand, is another matter.
Many manufacturing and industrial facilities have chosen to recruit capacity from a public cloud service provider to help them manage this data. However, while the cloud is a relatively cost effective, simple to manage, and scalable solution to suit your needs, it can also create some other challenges.
Why pushing everything to the cloud is not the answer
The cloud offers the enormous benefits of scalability without having to invest in on-premise data centre infrastructure and the IT resources to maintain it. On the other hand, cloud services do bear a cost that will climb as greater capacity or computational resources are used.
If you’re only looking to receive low-resolution data on the cloud (collecting only one measure value, like temperature, for example), you may be able to get away with a simple cloud architecture and still be able to access all the data you need in production real-time.
But if you want your system to receive higher resolution data and collect enough detailed information that would enable you to be able to perform true root cause analysis later (collecting and storing full waveforms, images—all the data from every process and not just the results), then this can become more of a costly, cumbersome cloud solution.
How can adding intelligence at the network edge help?
The goal is to pre-qualify and filter what data is sent to the cloud, and for what purpose, at the network edge.
We define edge computing as putting the capability to transform or translate what a sensor on a machine or process is telling us, as close to that sensor as possible. This means having a distributed network of intelligent nodes, or modules, paired with sensors. These modules are the gatekeepers that decide what to pass on to the cloud and what not to.
Consider it a needle and haystack exercise. What we want to spotlight are the needles – the data that matters. Why upload the entire haystack to the cloud when all you need are the needles – the data that is meaningful?
A node on the network edge can also do far more than just sort the needles from the straw. It can also perform real-time signal processing and analytics on all the data, to create transformed and aggregated information sets.
This filtered output can then be passed on to the cloud for storage and further analytics with other data. Cloud resources are used only to carry out the highest level of data storage and analysis, for operations such as root cause traceability, and to trend the performance and efficiency of an entire plant for continuous improvement.
In this way, latency issues are eliminated because the cloud is used only for the highest value operations.
Looking to add intelligence and edge analytics to your network?
The Sciemetric EDGE platform offers a compact, modular design the delivers in-depth insight into the performance, reliability and repeatability of a broad range of applications. Systems are remotely configurable for centralized management of your operations. Contact us to discuss how this platform could be used to improve your industrial operations.